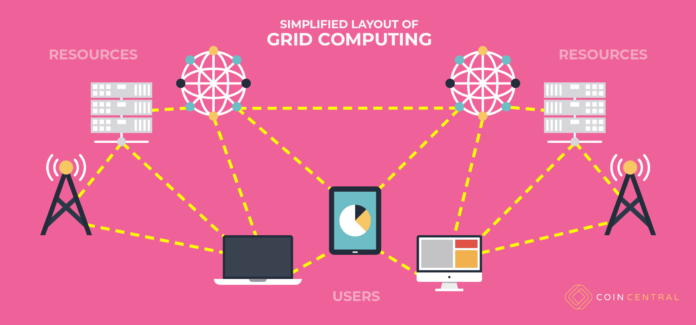
Grid computing, a descendant of the cloud and big brother to distributed computing.
Think of grid computing as the intersection of two core systems of organization: cloud computing and public utilities like electricity. At this intersection, grid computing is enabling you to tap into computational resources, centralized and not. Just like you would tap into the nearby energy lines for some of those glorious electrons that we rely on.
A modern power grid will have many sources of input. Power plants, for example, contribute a lot to the power grid but burgeoning technologies, such as solar panels and windmills, are democratizing power production.
Independent and artisanal power producers can contribute to the power grid and receive compensation. In some cases, this is excess energy.
Farmers, for example, may have solar panels to generate cheaper electricity locally. However, the farmer cannot store any unused electrons for future use, so they may choose to route that surplus energy back to the energy grid, where others can use it. One person’s wasted electrons are another’s fully charged Tesla.
Grid computing is much like the electricity grid. Contributors, big and small, can add to the grid. Users can tap into the computational grid and access services independent of the contributor.
The Cloud, Grid, and Distributed Computing
To better understand what grid computing is and its nuanced differences from distributed computing, it will be easier to first understand the barrier and limitations that grid computing is able to overcome. In other words, seeing the problems grid computing can solve will help us better understand what grid computing is.
The Limits of Cloud Computing Is Where the Grid Shines
Grid computing is a subset or extension of cloud computing. In a nutshell, cloud computing is the outsourcing of computational functions. A common cloud service, like cloud data storage from Google Drive or Dropbox, lets a customer store their data with those companies.
Someone looking to use cloud data storage chooses between providers like Google Drive, Dropbox and iCloud. The company they go with would then be their provider of cloud storage. Customer support, troubleshooting, billing, networking infrastructure, and all aspects to providing the cloud service to the customer would then come directly and solely from the it managed services company they choose for their cloud services.
Pretty straightforward, right? One customer, one provider. However, we are looking for the limitations of cloud computing. Where do the perks of cloud computing fall short and leave room for other organizational structures like grid computing?
Common Criticisms of Cloud Computing:
- User resources are committed to a single symmetric multiprocessing (SMP) system.
- Unused computing resources sit idle and are locked into a single task until it is complete.
- Relatively limited scalability.
Evolving Cloud Limitations with Grid Computing
Keeping in mind the parallels that grid computing has with a public utility grid, this type of computational organization can alleviate some of the common criticisms limiting cloud computing.
Let’s look over each of these claims and examine how a grid system could be more beneficial for a user over a traditional cloud service.
Cloud Limitation #1: User resources are committed to a single symmetric multiprocessing (SMP) system.
I’ll use a really basic example to showcase this pain point. There is a neural scientist looking to crunch two data sets (Set A and Set B). These data sets are huge and she’ll need to outsource the task to a cloud service.
The cloud service will have no problem running these data sets and you happily rent one machine from them to process her datasets. Remember that her datasets are exclusive to each other and need to be processed separately.
This means that the single SMP machine she leased will run Set A followed by Set B. Her single machine is unable to process both data sets simultaneously.
No big deal though, the cloud machines she leased are heavy duty and tear through the massive data sets in less than a few hours each. Processing the data will take less time than a full nights sleep for the scientist.
Now, what happens if she needs to do the same processing but for 100 data sets. Her budget still only gives her enough funding to access one cloud SMP machine. Being a person of science, she quickly does the math and discovers that it will take nearly two weeks to process all that data!
Grid Advantage: The same scientist with two data sets (Set A and Set B) could instead tap into a grid service. Instead of the scientist renting a single SMP machine from a cloud service, she would access the computing grid and rent the necessary computational power required.
The two data sets get processed at the same time. Perhaps by two machines, each dedicated to either data set, or it could be thousands of machines each fractionally processing the data sets. Regardless, the data is being processed parallel to each other. What took six hours before in two batches, now takes three hours in a single batch.
One hundred data sets? In theory, this would still only take three hours as each data set is processed side by side.
Cloud Limitation #2: Unused computing resources sit idle and are locked into a single task until it is complete.
Expanding on the above example of a neural scientist, the cloud service she leased independently processed her datasets, one after the other.
While processing either data set, the scientist noticed her rented hardware is only operating at 80 percent of it’s capacity. The remaining 20 percent is not enough to process the second data set, instead, it sits idly waiting for the next task.
Grid Advantage: The commodification of processing power allows a single task to be conducted across multiple machines. In the case of the scientist’s datasets, a grid system could process the data in a range of combinations between machines.
For example, the two datasets are allocated to two machines in the grid, each using 80 percent of the machine they are being processed on. The remaining 20 percent wouldn’t sit idly, instead, another user of the grid captures it. This use of idle capacity is an important component of the strengths of grid computing.
Cloud Limitation #3: Relatively limited scalability
There’s no denying that the capabilities of cloud computing are exponentially larger than most localized machines. The multiple layers to the cloud stack have enabled many more participants to the entire field than ever before possible.
Furthermore, cloud computing has many scaling benefits compared to self-custodianship of these same services. So to say that cloud computing is also limited in scalability may seem a touch paradoxical.
However, relative to cloud computing, scaling on a grid is even more achievable. This is in part due to the modularity of grid computing in addition to the more efficient use of idle resources.
Grid Advantage: Regardless if you are contributing to it or using it, scaling your task in a grid computing system can be as easy as installing a grid client on additional machines.
In the case of the neural scientist, she was able to scale her needs from two data sets to 100 data sets in the same timeframe, under the same budget.
Distributed Computing or Grid Computing?
Both! Well, sort of.
In conversation, it’s pretty common to use grid and distributed interchangeably. Fundamentally, both terms refer to fairly similar concepts. They are both systems for organizing and networking computational resources.
However, if you really want to split hairs, grid computing is the overall collection of distributed networks. Grid computing itself is a distributed network of distributed networks. Meta enough for you?
What’s Next for Grid Computing
This has been a very macro understanding of grid computing. In actuality, is a multifaceted system for organizing a range of dynamic and individual parts, in order to get the most out them. Each component of the computing grid is layered with complexity and utility, not unlike the multiple pieces required in a public power grid.
Similar to a public utility, how it works is a beast of its own. However, the real impact is the overall accessibility. Because, like a public utility, grid computing is increasingly becoming a plug-and-play service.
The next evolution of grid computing is likely in the blockchain. Grid computing relies on multiple stakeholders trusting each other. Already, projects like Cosmos Network are creating decentralized grid systems that foster network interoperability and leverage the powers of a grid computing network.
The post Grid Computing | The Powers of Distributed Cloud Computing appeared first on CoinCentral.
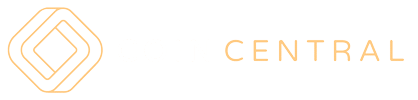
Coincentral.com is author of this content, TheBitcoinNews.com is is not responsible for the content of external sites.
Our Social Networks: Facebook Instagram Pinterest Reddit Telegram Twitter Youtube