The logarithmic rate of advancement of internet related technologies, machine learning science and techniques of data mining have led to wide application on the internet page information pattern analysis problems. A couple of researchers from Hebei University, China, have just proposed a new algorithm for deep web data mining in an attempt to boost the efficiency of currently present mining algorithms. The newly proposed deep web data mining algorithm relies on multi-agent information system along with a collaborative correlation rule. The proposed Multi-agent Information System (MAS) is comprised of several agents and uses parallel distributed processing technology as well as modular design thought, yet this complex system is divided into relatively independent subsystems of agents. The AdaBoost method is also combined with MAS to formulate the collaborative correlation rule. This combination produces an efficient and enhanced algorithm for deep web data mining. Performed experiments proved the feasibility of the proposed approach when compared with present deep web data mining approaches.
An Overview of the Newly Proposed Deep Web Data Mining Algorithm:
Deep web data mining represents the unknown dug up information extracted from a deep web page and the process of deciding whether or not the extracted information is potentially useful. The new approach ensures the following conditions:
- Mining web content: the approach will search through both text and non-text data sources such as databases, images, multimedia, graphics..etc. The algorithm explores structured data extracted from databases as well as useful HTML and XML tags, i.e. semi-structured data.
- Mining web use: records of usage of web servers , user access logs and users’ browsing information are mined through the algorithm whenever possible to predict patterns of user behavior to formulate a more predictive analysis.
- Mining of web structure: the algorithm also mines the organizational structure of links across the same website and between various websites. This data can be used to guide page classification and page clustering to discover authoritative pages in order to improve the process of retrieval of information.
The following figure represents the architecture of the web data pattern mining system.
Users browse the internet, with specific information needs in mind, and then click on a hyperlink to guide them through accessing content of a web page. The provided information has to be interesting in a way or another. If implicit information can be discovered in a user’s browsing path along the process of demand analysis, we can search through users’ interests and use the extracted data to provide search results that exactly fit the users’ needs. The new approach uses Markov’s prediction method which represents a method to estimate the probability of occurrence of a given event on basis of the Markov model, according to the current status events, to estimate the state of every future moment or period using the following predictive equation:
P(q1 = sj| qt-1 = si, qt-2 = sk ,….)
The utilized hidden Markov model represents a dual random process, with observation and state sequences. The state of state transition sequence probability, which also represents the process of model conversion is hidden and observable events of the stochastic process via the aforementioned analysis can use the HMM model.
Although the process of internet browsing represents a browse purpose process, cultural backgrounds, social levels, hobbies and other features often influence the overall process. Despite the fact that there exists great differences among various people, observation of a large number of people’s browsing patterns usually yields the same characteristics e.g. they basically browse the same web pages and the order of the pages browsed is highly similar. Consequently, this inspired the emergence of web user classification. Web user classification is very useful in deep web data mining and simplifies the process of extraction of useful information. The following equation defines the feasibility tool of measurement for the final evaluation:
Wp1 (rj) = (fji / fmax) / 2
Knowledge reasoning can be achieved efficiently in artificial intelligence via means of backward inference using association of reasoning rules, description of the association rules that show the basic relationship that exists between the URL support and the primary confidence of the quantitative standard which also represents the certain probability measure.
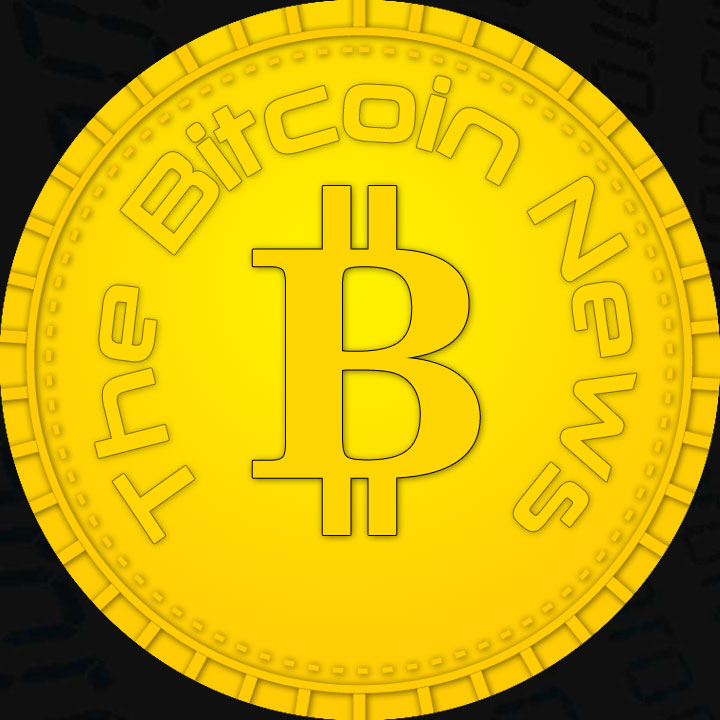
TheBitcoinNews.com – Bitcoin News source since June 2011 –
Virtual currency is not legal tender, is not backed by the government, and accounts and value balances are not subject to consumer protections. TheBitcoinNews.com holds several Cryptocurrencies, and this information does NOT constitute investment advice or an offer to invest.
Everything on this website can be seen as Advertisment and most comes from Press Releases, TheBitcoinNews.com is is not responsible for any of the content of or from external sites and feeds. Sponsored posts are always flagged as this, guest posts, guest articles and PRs are most time but NOT always flagged as this. Expert opinions and Price predictions are not supported by us and comes up from 3th part websites.
Advertise with us : Advertise
Our Social Networks: Facebook Instagram Pinterest Reddit Telegram Twitter Youtube